I’ve known Twyman’s law when I was in college. But not until I started working did I realize the importance of it.
The most common mistake analysts make is aiming to find different, interesting insights and immediately share them with the stakeholders afterward. All the excitement — can you believe our free trial users doubled in a week?! But more often than not, those insights are wrong either due to system bugs or calculation errors. That is Twyman’s law.
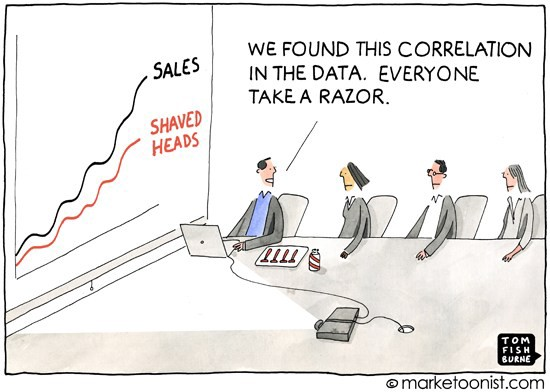
Definition
To give it an official definition, Twyman’s law is the principle that “ the more unusual or interesting the data, the more likely they are to have been the result of an error of one kind or another” (Wikipedia), meaning any metric or stats that looks interesting or different is usually wrong. If anything is too good/bad to be true, it’s probably not true.
Implication
“Extraordinary claims require extraordinary evidence.”— Carl Sagan
The practical implication is that you should be alerted when interesting findings appear and double-check them more carefully than regular ones.
For example, if an experiment’s result differs significantly from many similar ones before, investigate if the setup was correct, if the right data was collected, and if the calculation was accurate before wrong decisions are made from the “interesting” finding.
Twyman’s law is one of the most important laws for data analysis that it should be every analyst’s mantra.